Publications
Neurons are typically sensitive to a small fraction of stimulus space. If the environment changes, making certain stimuli more prevalent, neurons sensitive to those stimuli would respond more often and therefore have a higher average firing rate if the stimulus-response mapping remains fixed. However, sufficiently prolonged exposure to the new environment typically causes such neurons to adapt by responding less vigorously. If adaptation consistently returns the average firing rate of neurons, or populations of similarly tuned neurons, to its value prior to environmental shift, it is termed firing-rate homeostasis. Another feature of adaptation in sensory cortex is stimulus specific adaptation, under which neurons not only adapt their responsiveness, but also reshape their tuning curves away from overrepresented stimuli. Here, we present a normative explanation of firing-rate homeostasis grounded in the efficient coding principle. Unlike previous theories based on efficient coding, we formulate the problem in a computation-agnostic manner, enabling our framework to apply far from the sensory periphery. We show that homeostasis can provide an optimal solution to a trade-off between coding fidelity and the metabolic cost of neural firing. We provide quantitative conditions necessary for the optimality of firing-rate homeostasis, and predict how adaptation should deviate from homeostasis when these conditions are violated. Based on biological estimates of relevant parameters, we show that these conditions do hold in areas of cortex where homeostatic adaptation has been observed. Finally, we apply our framework to distributed distributional codes, a specific computational theory of neural representations serving Bayesian inference. We show that the resultant coding scheme can be accomplished by divisive normalisation with adaptive weights. We further demonstrate how homeostatic coding, coupled with such Bayesian neural representations, explains stimulus-specific adaptation, as observed, e.g., in the primary visual cortex.~
When stimulated, neural populations in the visual cortex exhibit fast rhythmic activity with frequencies in the gamma band (30-80 Hz). The gamma rhythm manifests as a broad resonance peak in the power-spectrum of recorded local field potentials, which exhibits various stimulus dependencies. In particular, in macaque primary visual cortex (V1), the gamma peak frequency increases with increasing stimulus contrast. Moreover, this contrast dependence is local: when contrast varies smoothly over visual space, the gamma peak frequency in each cortical column is controlled by the local contrast in that column’s receptive field. No parsimonious mechanistic explanation for these contrast dependencies of V1 gamma oscillations has been proposed. The stabilized supralinear network (SSN) is a mechanistic model of cortical circuits that has accounted for a range of visual cortical response nonlinearities and contextual modulations, as well as their contrast dependence. Here, we begin by showing that a reduced SSN model without retino- topy robustly captures the contrast dependence of gamma peak frequency, and provides a mechanistic explanation for this effect based on the observed non-saturating and supralinear input-output function of V1 neurons. Given this result, the local dependence on contrast can trivially be captured in a retinotopic SSN which however lacks horizontal synaptic connections between its cortical columns. However, long-range horizontal connections in V1 are in fact strong, and underlie contextual modulation effects such as surround suppression. We thus explored whether a retinotopically organized SSN model of V1 with strong excitatory horizontal connections can exhibit both surround suppression and the local contrast dependence of gamma peak frequency. We found that retinotopic SSNs can account for both effects, but only when the horizontal excitatory projections are composed of two components with different patterns of spatial fall-off with distance: a short-range component that only targets the source column, combined with a long-range component that targets columns neighboring the source column. We thus make a specific qualitative prediction for the spatial structure of horizontal connections in macaque V1, consistent with the columnar structure of cortex.
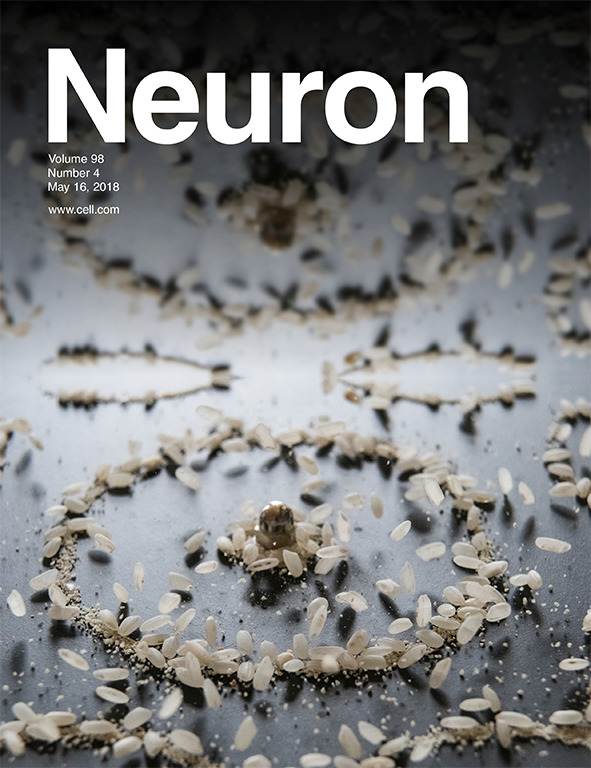
balanced network'', which yields only linear behavior. We more exhaustively analyze the 2-dimensional case of 1 excitatory and 1 inhibitory population. We show that in this case dynamic stabilization will occur whenever the determinant of the weight matrix is positive and the inhibitory time constant is sufficiently small, and analyze the conditions for
supersaturation’’, or decrease of firing rates with increasing stimulus contrast (which represents increasing input firing rates). In work to be presented elsewhere, we have found that this transition from supralinear to sublinear summation can explain a wide variety of nonlinearities in cerebral cortical processing.